article
To be a leader in AI, Canada must remove roadblocks
This article was originally published in The Gazette.
Affordable energy storage, lighter planes and cures for cancer could all be discovered through computational methods, given sufficient data. The recent Nobel Prize in Physics awarded to British-Canadian Geoffrey Hinton for his groundbreaking work on neural networks underscores AI’s transformative potential in scientific discovery. This recognition celebrates Canada’s contributions to AI and highlights the critical importance of computational resources in advancing science and technology.
However, a growing gap exists between Canada’s current high-performance computing resources and the computational needs of cutting-edge AI research. The Digital Research Alliance of Canada oversees the nation’s public high-performance computing resources, managing five national clusters worth millions.
While these resources adequately met demand in the early 2010s, they have since fallen drastically behind. Allocation results reveal a growing divide between available resources and the explosive growth in demand, particularly in the latter half of the decade. This year, only 40 per cent of the demand was met.
The situation is even more critical for graphical processing units (GPUs) — the hardware powering AI — where only 15 per cent of demand was met, and much of the hardware is over eight years old. This poses a major roadblock for modern, demanding tasks. This growing disparity highlights the urgent need for infrastructure upgrades across Canada’s research institutions. Universities with deeper pockets could gain an unfair advantage by purchasing their own systems.
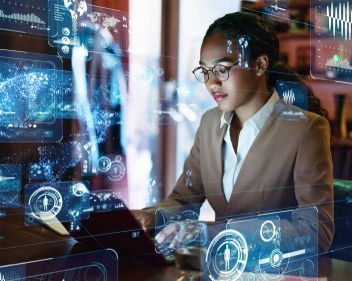
While all researchers receive basic access to the computing infrastructure, they face extended wait times due to lower priority status, sometimes spanning days for larger tasks. This delay significantly hampers research productivity, especially for students. Scientists must strategically focus their efforts within limited resources.
Without adequate resources, scientists may miss uncovering the next cure for Alzheimer’s or breakthrough battery material. Improved infrastructure would broaden research scope, enhancing scientific output quality and impact. The data generated would feed AI prediction models, potentially accelerating the transition from laboratory discoveries to industrial applications.
In recent years, scientists have been collecting vast amounts of data for AI applications. These improved AI models can predict effective materials or molecules, without the need to synthesize them in the lab first, saving time and money. An example is the recent breakthrough by University of Toronto researchers, led by professors Christine Allen and Alán Aspuru-Guzik. Their team employed machine learning to reduce drug development time. These scientists assert that AI models need large amounts of data to perform well.
However, some niche research areas lack sufficient data. This underscores the importance of teams of computer experts who can create and share the necessary data. It also highlights how data collection, AI modeling and laboratory experiments work together to drive scientific progress and innovation. Critics favour private-sector AI development, but compute costs can be prohibitive, especially for startups.
A 10-person research team could face over $20,000 in annual computing costs, according to estimates from the Digital Research Alliance. This issue is particularly pressing in Canada, where computing infrastructure lags behind many developed nations. Publicly funded computing infrastructure could alleviate costs for smaller entities, while larger businesses could pay for access. Finland’s model reserves 20 per cent of supercomputer capacity for industry partners.
This approach encourages private-sector involvement in AI development while reducing public costs. It also fosters innovation by providing computer access to both small and large entities. Canada must provide the means to generate data in a world where it is a valuable commodity. Robust AI infrastructure is crucial for empowering entrepreneurs, researchers and businesses to develop specialized AI models.
Without adequate resources, Canada risks losing its talented experts and innovative startups to countries offering not only higher compensation but also superior computational power — both essential for cutting-edge AI development and applications. As Hinton’s Nobel Prize demonstrates, Canada has the potential to lead in AI innovation; now we must ensure we have the computational foundation to support the next generation of groundbreaking discoveries.
Ameer Nizami is a PhD candidate and public scholar at Concordia University, researching batteries with AI.