News release
NEW RESEARCH: This automated system could reduce metro repairs and save inspection time
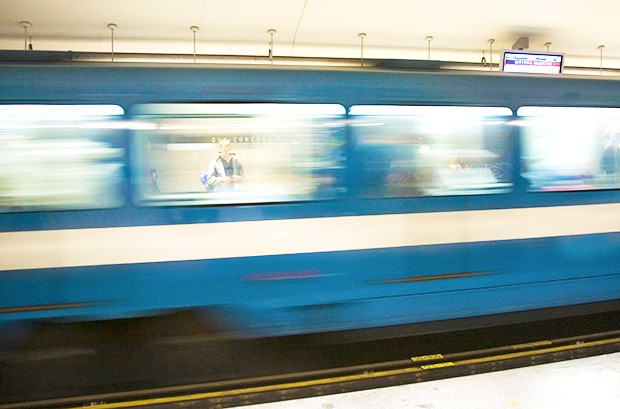
Montreal, September 11, 2017 - Urban infrastructure managers and civil engineers now have a better tool to detect and evaluate structural distress in subway networks, thanks to Concordia’s Department of Building, Civil and Environmental Engineering (BCEE).
PHD candidate Thikra Dawood, in collaboration with professor Tarek Zayed and assistant professor Zhenhua Zhu, has developed an integrated model for the detection and quantification of spalling, a significant surface defect in concrete infrastructure. Left untreated, it can compromise integrity and lead to collapse. Their new research was featured in an article recently published in Automation in Construction.
The model integrates digital photography, image-processing techniques and computer-based learning in a largely automated process. It is being touted as a more objective and consistent substitute for the visual techniques typically used by inspectors to assess spalling distress on-site.
Research has found the latter methods to be time-consuming, costly, labour-intensive and error-prone.
Subjective distress
Spalling is typically caused by water infiltration and can be identified by the telltale pitting of concrete surfaces. The depth of the pitting correlates with the severity of the flaw in the structure, but the severity is difficult to diagnose by visual means alone.
“Visual inspection techniques are the most common form of identifying spalling distress, but they’re not reliable in terms of accuracy,” says Dawood, the article’s lead author.
“If you send two inspectors to the same site, they’re liable to produce divergent measurements of the distress. This is because their methods are subjective, based on their knowledge and experience. What’s more, some places are inaccessible to the inspectors, and they’re forced to rely on estimates.”
In place of visual techniques, Dawood’s integrated model proposes a simple three-step process: first, an image of the spalling defect is taken with a digital camera. Then the image is transferred to a computer, where it is treated with image-processing algorithms and filters to remove the noise within its frame and separate the defect from the background of the image.
Finally, the enhanced information detected within the image is channeled to a regression model and developed using a programming language known as MATLAB.
“At this stage, we can determine the length and width of the defect, which in turn can be used to create a realistic 3D model of the spalling defect.”
Testing in Montreal’s underground
In order to implement the model, Dawood sought and received permission from the Société de transport de Montréal to study the city’s subway network. She worked primarily in a station on the orange line with high rates of congestion and various signs of surface deterioration.
Working in the early morning hours, Dawood took a total of 75 images of various spalling defects in the station using a high-resolution camera. She then measured the actual depths of the defects with a measuring tape and a steel plate.
By placing the plate over the defect, she could pass the measuring tape through to determine the depth of each point in the depression.
Later, Dawood developed the algorithms for treating the images. She also developed a regression analysis to compare the pixel intensity levels of the refined images to the actual recorded depths of the defects. She then used this data to find a model that could reliably project depth distress.
In the end, the detection ability of the image-processing algorithm was validated for all 75 images. It attained 91.7 per cent recall, 94.8 per cent precision and an overall accuracy of 89.3 per cent. In addition, the regression analysis was able to satisfactorily quantify the depth of spalling with an average validity of 93 per cent.
‘Automation will drastically reduce unnecessary work’
Dawood sees the model as one positive step toward greater automation in subway infrastructure inspection techniques.
“Everything is developing and progressing at such a fast pace these days,” she says. “I think automation will drastically reduce unnecessary work in our lifetime. It’s the future.”
Dawood has already used the model to assess other types of surface defects, such as cracks and moisture marks. The ultimate aim is to develop a generic condition assessment model for subway networks.
As it stands now, the STM has expressed a strong interest in her model.
“I’d love to see them adopt it,” Dawood says.
Read the cited study.
Source
© Concordia University