Amir Molaei is a doctoral candidate in Mechanical Engineering. He holds an MSc degree in Mechatronics and a BSc in Mechanical Engineering both from K.N. Toosi University of Technology, Tehran, Iran. Amir's research is focused on the design, modelling and control of flexible robots to help surgeons operate inside the eye in a more precise and a less invasive way.
Blog post
Artificial intelligence as a doctor for the diagnosis of eye diseases
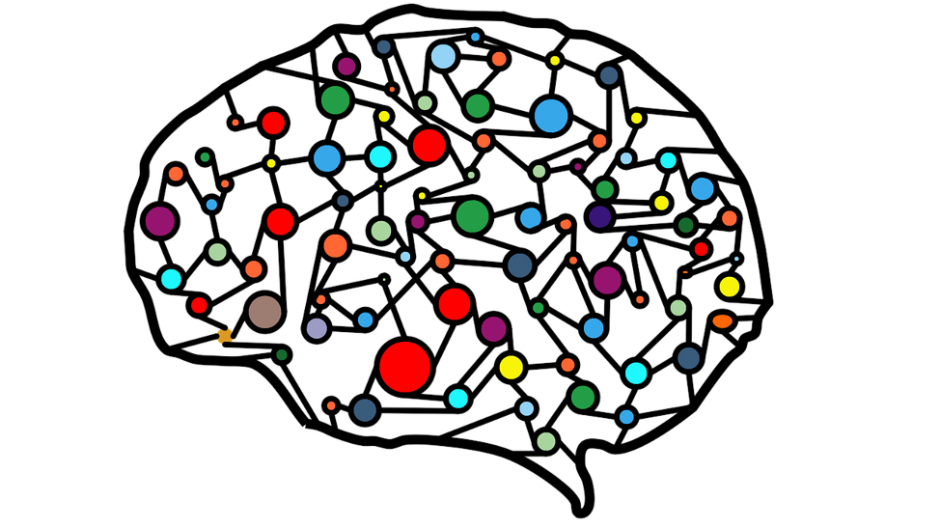
Artificial intelligence (AI) and its subsets has shown great promise to increase health care affordability, quality and accessibility. In the early diagnostic stages of eye diseases, the complexity of medical images exceeds the ability of humans to interpret this information. At the same time, the world suffers from shortages of expert ophthalmologists.
However, newly developed methods in AI, such as using deep learning (DL), has led to new techniques for improved speed and accuracy of screening. The ability of DL systems in some cases is beyond that of an expert doctor and can even go further to make referrals for various eye conditions. Experts have already used DL techniques to help diagnose macular oedema and age-related macular degeneration and diabetic retinopathy.
What follows is a glimpse into how these diseases can be threatening for human sight and how AI will come to help us in this regard.
Diabetic retinopathy
Diabetic retinopathy (DR) is a complication from diabetes that damages the blood vessels of the retina. At its early stages, there are no symptoms but it eventually can cause blindness. The extra sugar in the blood leads to blockages of vessels at the retina, and the eye tries to compensate for it by growing new blood vessels which are not fully functional.
DR is one of the primary causes of blindness and visual loss. By 2040, projections indicate that one-third of people with diabetes will develop diabetic retinopathy, which accounts for 200 million individuals.
Age-related macular degeneration
Age-related macular degeneration (AMD), which many refer to as ‘Alzheimer's of the eye,’ occurs due to the damage to the retina and results in blurred or loss of vision in the centre of the visual field. AMD is the leading cause of severe, permanent vision loss in people 60 years and older. Projections indicate that AMD will impact 288 million people by 2040.
Glaucoma
Glaucoma is a leading cause of irreversible blindness worldwide. The eye disease slowly damages the nerves within the retina, causing irreparable harm before there is any vision loss. Many consider high intraocular pressure (IOP) to be the primary reason for glaucoma, which occurs when the eye drainage system does not function properly.
According to the World Health Organization (WHO), glaucoma is the second leading cause of blindness in the world after cataracts. In 2013, 64.3 million people had glaucoma, and experts estimate that those numbers could rise as high as 111.8 million by 2040.
By 2040, projections indicate that 600 million individuals will suffer from severe retinal diseases, which highlights the importance of effective screening methodologies and referral programs. Soon, AI will play a vital role to help eye doctors process medical images and classify retinal diseases and their severity to plan for suitable treatment.
How AI works for the diagnosis
Experts diagnose these eye diseases based on the processing of the medical images of the retina, including very large data which is hard for experts to analyze. One of the most effective machine learning approaches for image processing is DL, which draws inspiration from the human biological nervous system.
The development of DL algorithms allows for significant progress to classify images and detect objects in a picture. DL algorithms aim to mimic the human experience for the same task by discovering image features and comparing them to the normal condition to identify pathological classes. Experts can design AI systems to continuously adapt and improve over time as they receive and train with more data input.
A DL network consists of multiple sequential layers that take input, perform some processing on the input data, and forward the current layer output to the subsequent layer. Each layer has some parameters that need optimization based on the training algorithm of the network.
For training a DL network, we need to have a data set of retinal-labeled images — each image is pre-identified to be healthy or with a defect related to a specific disease. Experts will classify the data set images into two main categories — training sets (70 per cent data) and test sets (30 per cent data).
At first, preprocessing is done to remove the noise, normalize each color channel and make the size of the images equal. The training procedure is done by passing a batch of data to the network. At each training step the parameters of the network is tuned.
After training the network, the performance of the network is analyzed using test data. With suitable accuracy, the network can be used to classify any images to find eye problems.
Challenges
The power of DL algorithm lies in its capacity to process and encode big data, which at the same time is vulnerable to bias based on the training data. This makes the diversity in the data set very important in the training of the algorithm, with geographically and racially diverse settings.
Most of the notable research in this field have only evaluated their algorithms on subjects from the same populations as that of the training, and there is limited research in which the algorithm has been tested for the external data set, which has not been used in the creation of the algorithms.
Additionally, variations of imaging technology — different devices, noise and aging of the components — do still require exploration. Further work is needed to investigate the influence of imaging technology and image quality in the performance of algorithms.
As a ‘black box’ method, DL makes it hard for physicians to trust the decisions of AI methods to best match the clinical decision-making process. Additionally, in times of stress and uncertainty, many patients prefer interactions with their physician.
About the author
