Predicting microclimates: a new AI approach to urban sustainability
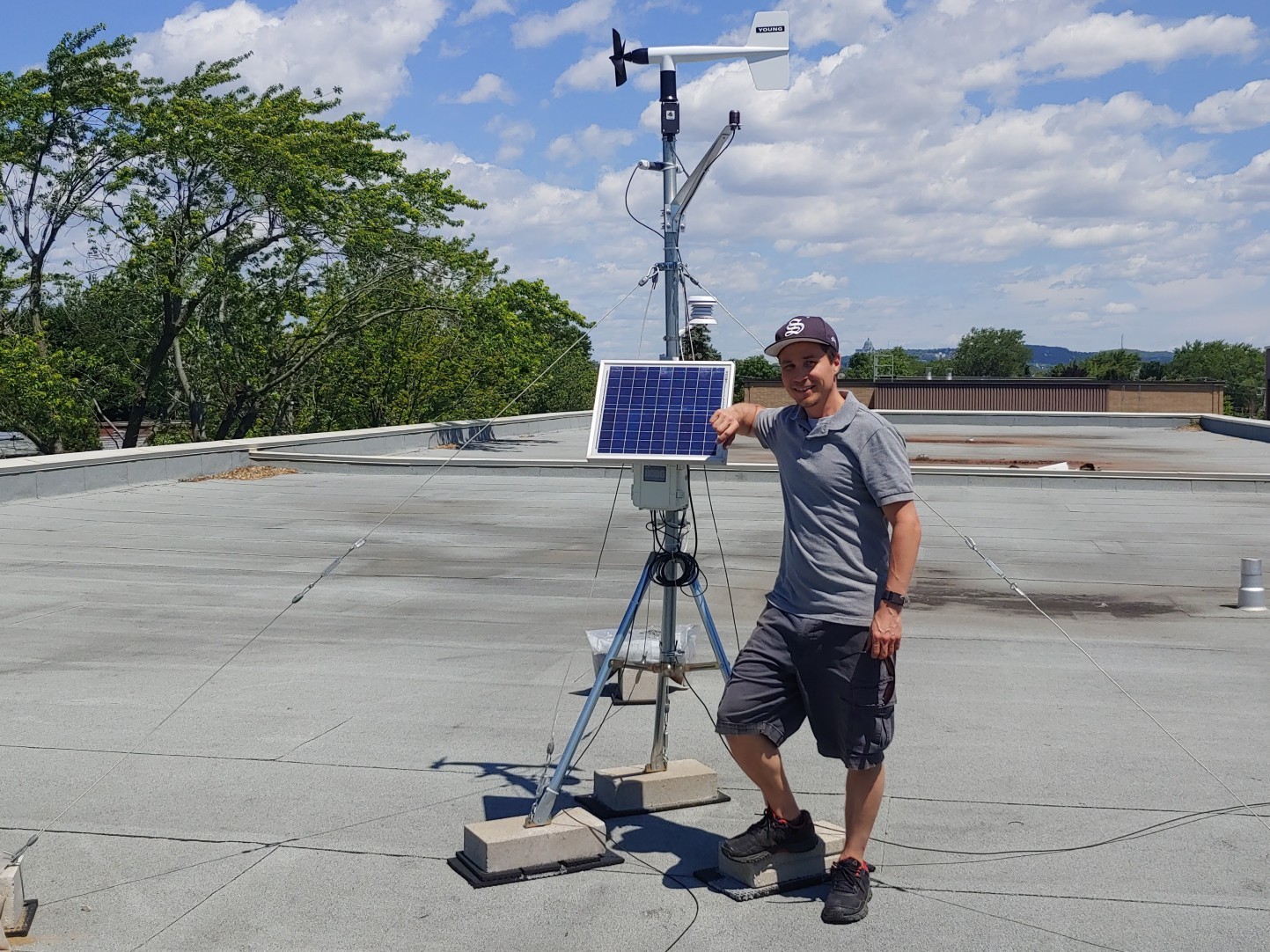
By 2050, it is projected that over 70% of the global population will reside in urban areas, which will face not only heightened energy demands but also increased frequency of extreme weather events due to climate change.
Addressing these challenges, PhD student Senwen Yang from the Concordia’s Department of Building, Civil and Environmental Engineering has pioneered a method using artificial neural networks (ANN) to enhance predictions of urban microclimates using data from local weather stations.
Guided by Gina Cody School of Engineering and Computer Science professors Leon Wang and Ted Stathopoulos of the Centre for Zero Energy Building Studies, Yang's innovative approach synthesizes 20-year long-term weather data from airports with several months of localized observations. From this, he has developed a model capable of generating an urban-specific typical meteorological year tailored to address the unique climate nuances of cities. This model was tested using data from five weather stations across Montreal.
The findings were enlightening: urban microclimates could potentially increase cooling energy consumption by up to 14% while reducing heating requirements by as much as 10%. Such insights could empower city planners and building managers to fine-tune heating and cooling systems, paving the way for more sustainable and eco-friendly urban environments.
For more detailed insights, refer to the full study titled “Urban microclimate prediction based on weather station data and artificial neural network,” published in Energy and Buildings.