Social media’s fake news problem is the target of a new tool developed at Concordia
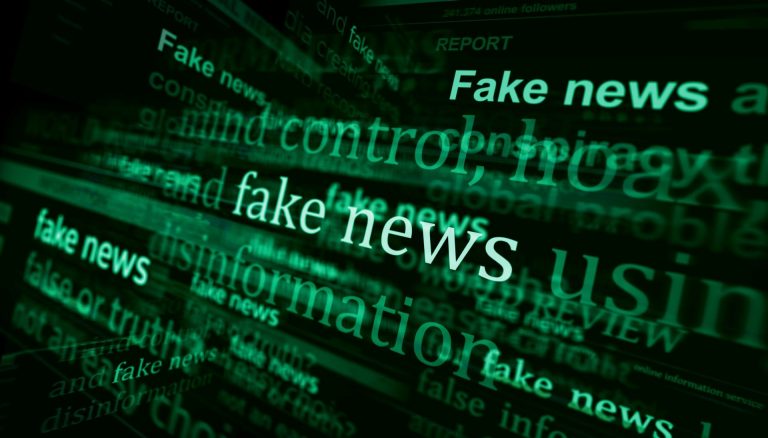
Fake news across social media is becoming ever easier to spread and more difficult to detect. That’s thanks to increasingly powerful artificial intelligence (AI) and cuts to fact-checking resources by major platforms.
This is especially concerning during elections, when local and international actors can use images, text, audio and video content to spread misinformation.
However, just as AI and algorithms can propagate fake news, they can be used to detect it. Researchers at Concordia’s Gina Cody School of Engineering and Computer Science have developed a new approach to identifying fake news. And they say it will be able to find hidden patterns that reveal whether a particular item is likely fake or not.
The model, called SmoothDetector, integrates a probabilistic algorithm with a deep neural network. It’s designed to capture the uncertainties and key patterns in the shared latent representations of texts and images in a multimodal setting. The model uses annotated text and image data from the United States–based social media platform X and the China-based Weibo to learn. The researchers are currently looking into ways to eventually incorporate functionalities to detect fake audio and video content as well, leveraging every medium to counter misinformation.
“SmoothDetector is able to uncover complex patterns from annotated data, blending deep learning’s expressive power with probabilistic algorithm’s ability to quantify uncertainty, ultimately delivering confident prediction on an item's authenticity,” says PhD candidate Akinlolu Ojo. He describes the model in the journal IEEE Access.
One of the complexities the model learns is tone. Positional encoding gives the model the ability to learn the meaning of a certain word in relation to others in a sentence, providing it with a coherence to the sentence. The same technique is used on images.
“The innovation of our model lies in its probabilistic approach,” Ojo says.
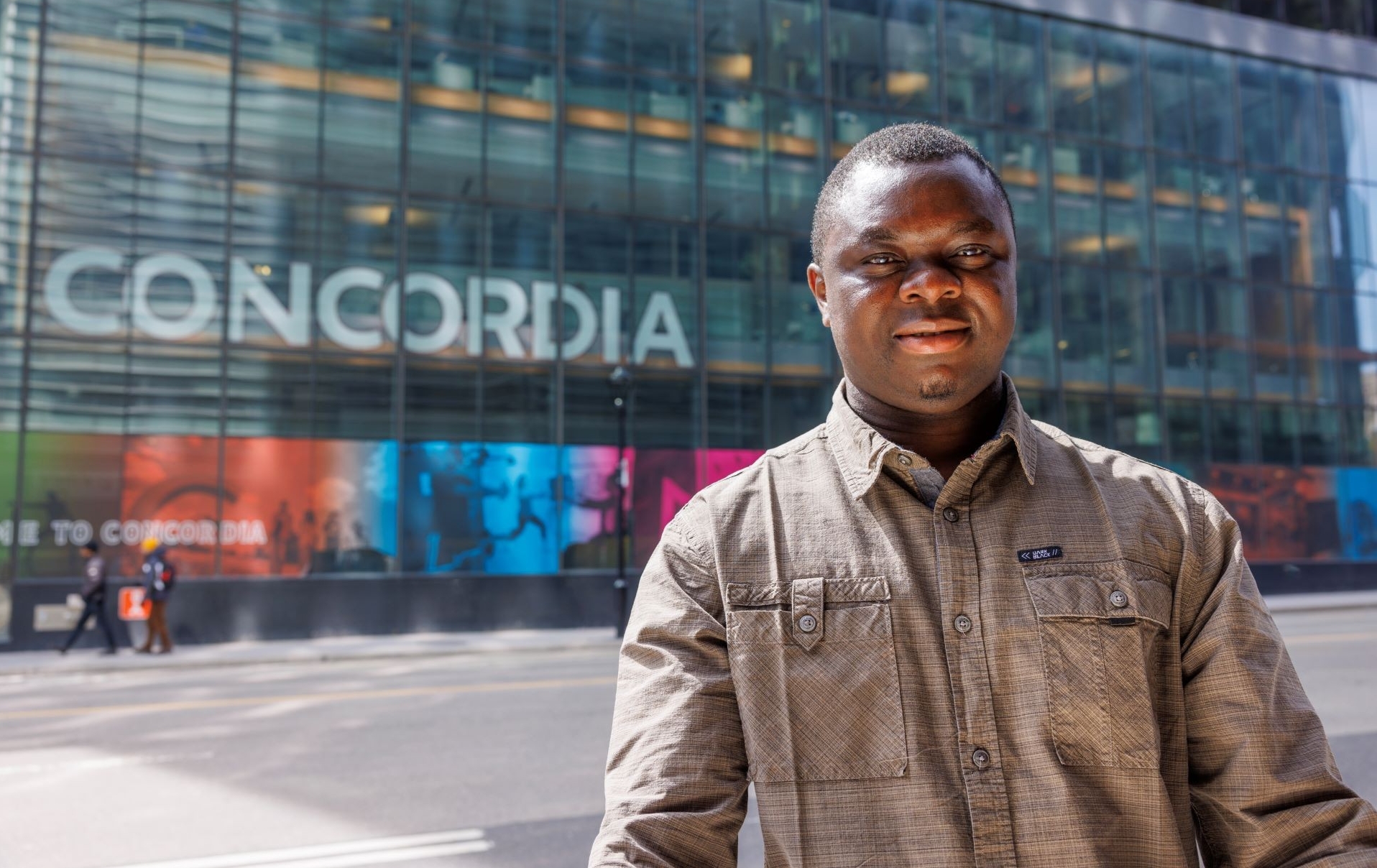
Learning possible ambiguity
SmoothDetector builds on existing though still relatively new multimodal models of fake news detection, Ojo explains. Earlier models could only examine one mode at a time — text or image or audio or video — rather than all modes of a post simultaneously. That meant that a post with fake text but an accurate photo could be labelled as a false positive or negative.
This could create additional confusion especially with regards to breaking news, when large amounts of information is generated quickly and can be contradictory.
“We wanted to capture these uncertainties to make sure we were not making a simple judgement on whether something was fake or real,” Ojo says. “This is why we are working with a probabilistic model. It can monitor or control the judgement of the deep learning model. We don’t just rely on the direct pattern in the information.”
SmoothDetector gets its name from the smoothing of the probability distribution of an outcome: instead of directly deciding that a piece of content is fake or real, it assesses the inherent uncertainty in the data and quantifies the likelihood to smooth the probability, offering a more nuanced judgement of an item authenticity.
“This makes it more versatile to capture both positive and negative information or correlation,” he adds.
Ojo says that although more work is needed to make the model truly multimodal and able to analyze audio and visual data, it is transferrable to other platforms besides X and Weibo.
Nizar Bouguila, a professor at the Concordia Institute for Information Systems Engineering, contributed to this paper, along with assistant professor Fatma Najar, PhD 22, at the John Jay College of Criminal Justice, with assistant professors Nuha Zamzami, PhD 20, and Hanen Himdi at the University of Jeddah in Saudi Arabia.
Read the cited paper: “SmoothDetector: A Smoothed Dirichlet Multimodal Approach for Combating Fake News on Social Media.”