New Concordia research shows social networks are vulnerable to relatively simple AI manipulation and polarization

It seems that no matter the topic of conversation, online opinion around it will be split into two seemingly irreconcilable camps.
That’s largely a result of these platforms’ design, as the algorithms driving them direct users to like-minded peers. This creates online communities that very easily become echo chambers, exacerbating polarization.
The platforms’ own vulnerabilities to outside manipulation make them tempting targets for malicious actors who hope to sow discord and unsettle societies.
A recent paper by Concordia researchers published in the journal IEEE Xplore describes a new method of making this easier. The approach uses reinforcement learning to determine which hacked user’s social media account is best placed to maximize online polarization with the least amount of guidance.
“We used systems theory to model opinion dynamics from psychology that have been developed over the past 20 years,” says Rastko Selmic, a professor in the Department of Electrical and Computer Engineering at the Gina Cody School of Engineering and Computer Science and a co-author of the paper.
“The novelty comes in using these models for large groups of people and applying artificial intelligence (AI) to decide where to position bots — these automated adversarial agents — and developing the optimization method.”
The paper’s lead author, PhD candidate Mohamed Zareer, explains that the goal of this research is to improve the detection mechanisms and highlight vulnerabilities in a social media network.
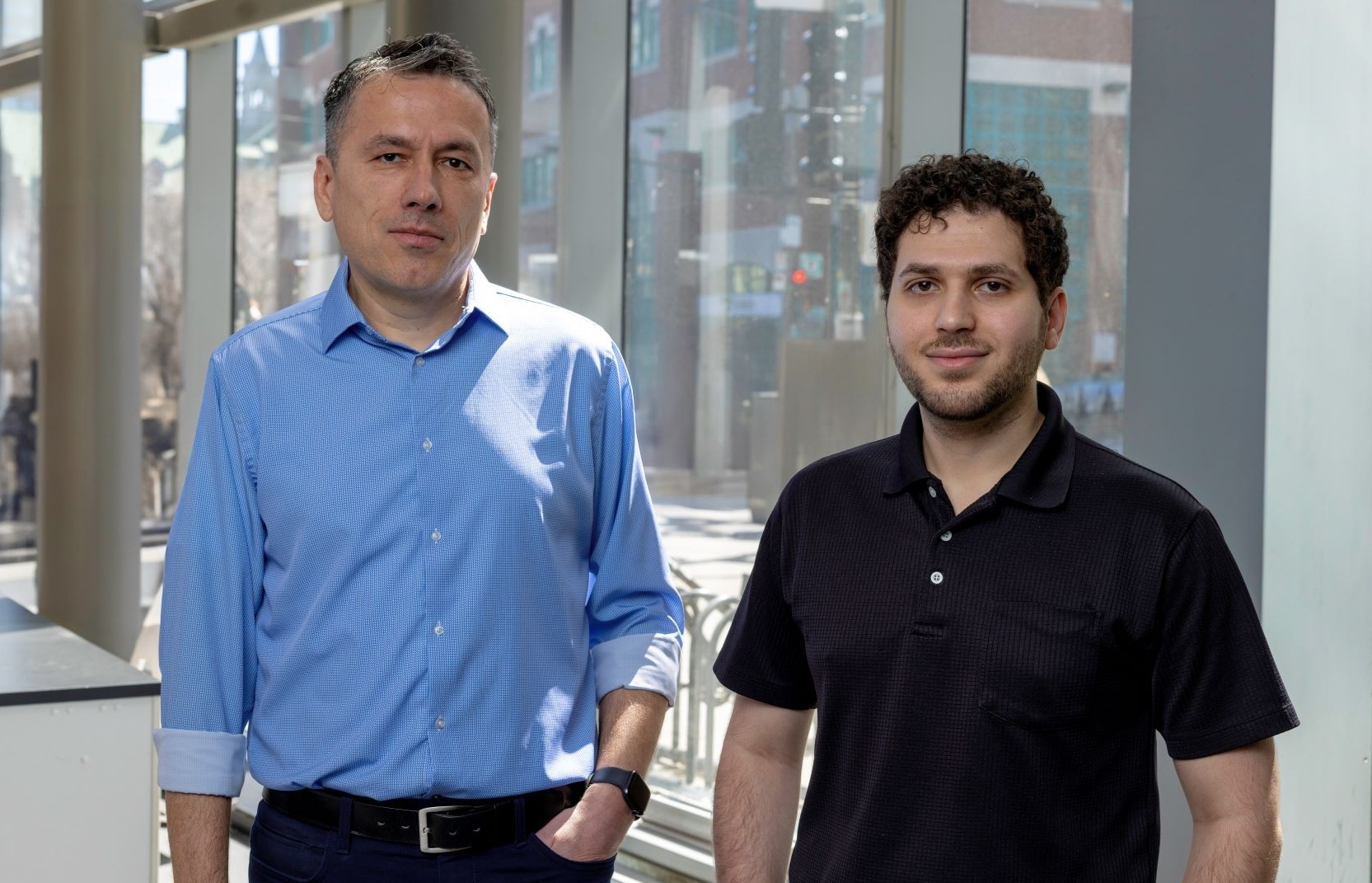
A little data can do a lot of harm
The researchers used data from roughly four million accounts on the social media network Twitter (now X) that had been identified as having opinions on the topic of vaccines and vaccination.
They created adversarial agents that used a technique called Double Deep Q-Learning. This reinforcement-learning approach allows bots to perform complex, rewards-based tasks in complex environments like a social media network with relatively little oversight by human programmers.
“We designed our research to be simple and to have as much impact as possible,” Zareer says.
In their model the adversarial agents would only have two pieces of information: the current opinions of the account owner and the number of followers. The researchers applied their algorithm to three probabilistic models that ran them through synthetic networks of 20 agents, which they say makes the results representative and generalizable.
These and other experiments mimic actual threats like bots or coordinated disinformation campaigns. They confirm the effectiveness in intensifying polarization and creating disagreements across social networks.
The researchers hope their work will influence policy-makers and platform owners to develop new safeguards against malicious manipulation by malicious agents and promote transparency and ethical AI usage.
Read the cited paper: “Maximizing Opinion Polarization Using Double Deep Q-Learning on Social Networks.”