Decoding sound: How new tech lets us “listen” to machine thoughts
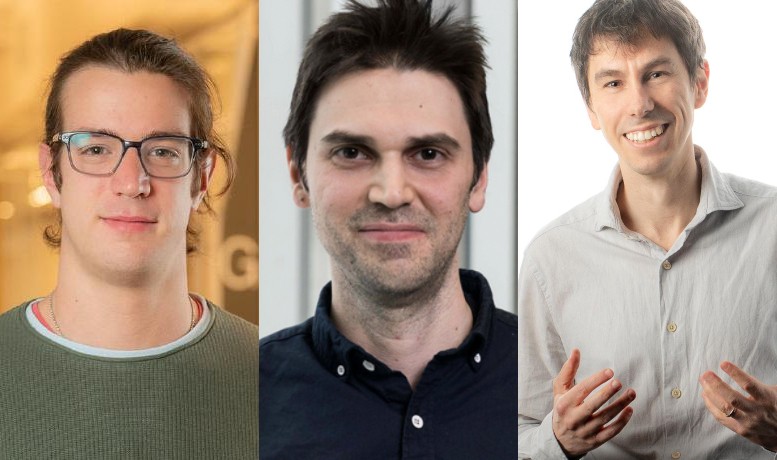
Every time you speak to your smartphone, or a voice-operated assistant accurately recognizes a command, there's complex technology at work. Understanding this technology is not just about convenience, it's about trust and transparency.
To address the challenge of interpreting how machines process sound, researchers have developed a new method called Listenable Maps for Audio Classifiers (L-MAC), which simplifies machine decisions by letting us listen to them.
L-MAC employs a special technique that highlights the crucial elements of sound influencing a machine’s decision-making process. This allows anyone to audibly experience why a machine made a specific choice, fostering greater transparency and trust in automated systems.
The method was developed by a team including:
- Lead author Francesco Paissan, a researcher at Fondazione Bruno Kessler and visiting researcher at Mila
- Cem Subakan from Université Laval and Mila, who is also an affiliate assistant professor at Gina Cody School’s Department of Computer Science and Software Engineering at Concordia
- Mirco Ravanelli, assistant professor in the Gina Cody School’s Department of Computer Science and Software Engineering at Concordia.
"L-MAC helps to understand the decisions made by complex, and ‘black-box’, neural networks by providing an explanatory signal that anyone can understand just by listening," explains Ravanelli.
Comparisons with previous techniques show that L-MAC provides clearer explanations of machine decisions, demonstrating its effectiveness through detailed side-by-side analysis.
A user study, involving real people interacting with the system to evaluate its performance and usability, showed that participants found L-MAC’s audio explanations more intuitive and accessible than traditional methods.
Their work was showcased during an oral session at the 41st International Conference on Machine Learning (ICML) in Vienna, Austria—a prestigious event where only 1.5% of submissions are selected for an oral presentation.
For more insights and to hear the sounds that influence machine decisions, read the full article in Proceedings of the 41st International Conference on Machine Learning and consult Paissan’s website. The code for the proposed technique has also been made publicly available in the open-source conversational AI SpeechBrain toolkit.
Learn more about the Department of Computer Science and Software Engineering at the Gina Cody School.