Gina Cody School researchers improve persistent cyber threat detection
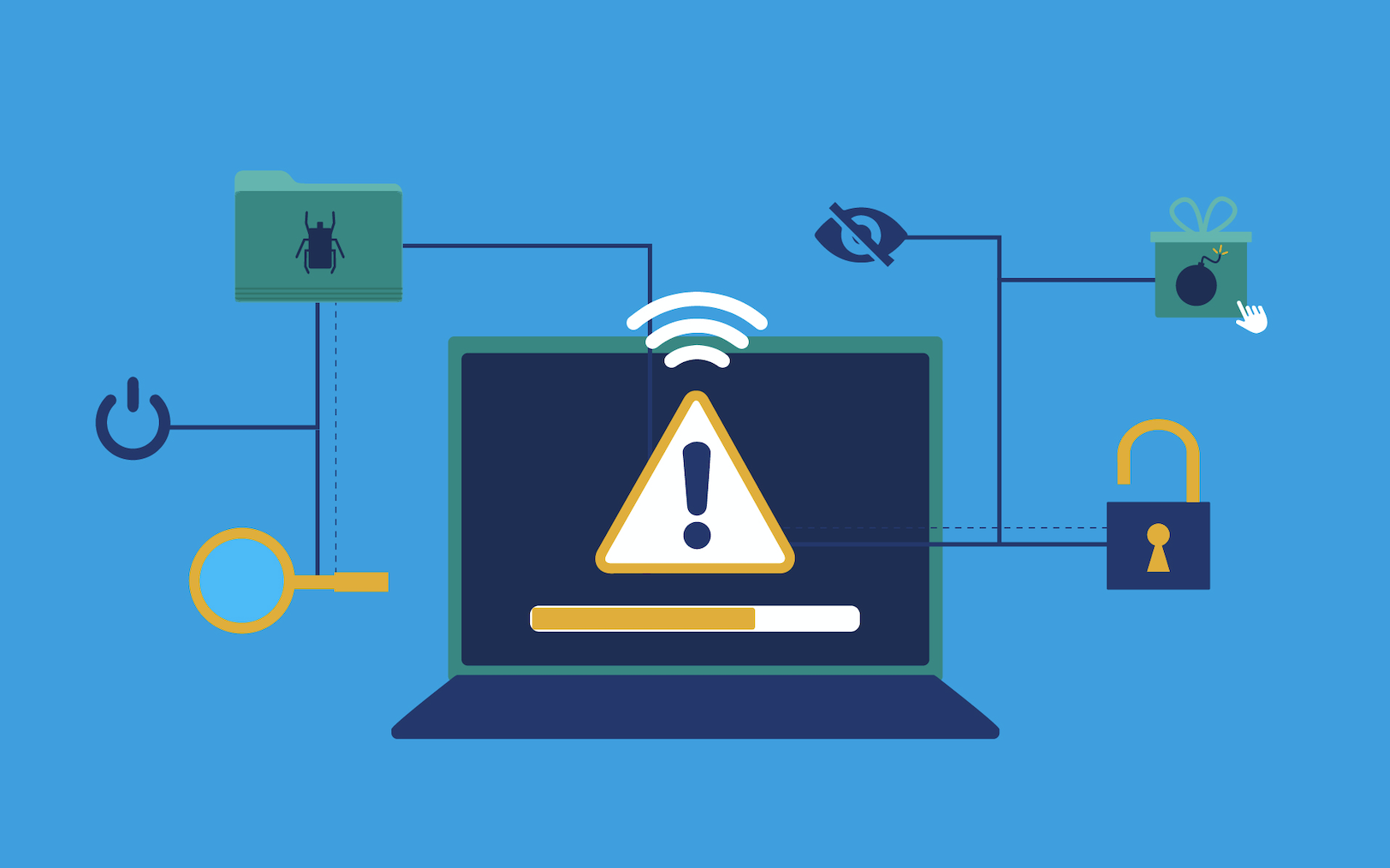
As cybersecurity threats are becoming more sophisticated and harder to detect, researchers at Concordia University’s Gina Cody School of Engineering and Computer Science have designed a new system to uncover hidden cyber threats called Advanced Persistent Threats (APTs), which can lurk in computer systems for months or even years without being detected.
Detecting these threats is like finding a needle in a haystack because they blend in with normal activities on computer systems.
To identify these stealthy threats more efficiently, PhD student Ahmed Aly and Professors Essam Mansour and Amr Youssef, in collaboration with Shahrear Iqbal from National Research Council Canada, introduce a new system, called MEGR-APT: Memory-Efficient Graph Representation of Advanced Persistent Threats.
"MEGR-APT has the potential to transform how we detect cyber threats,” explains Mansour who is a professor in the Department of Computer Science and Software Engineering. “By making detection faster and more memory efficient, we can better protect sensitive information and critical infrastructure from attacks."
“One of the biggest problems with current detection systems is that they either use a lot of computer memory or take too long to process data,” says Youssef, professor in the Concordia Institute for Information Systems Engineering. “We developed a smarter approach.”
MEGR-APT looks at the records of what happens on a computer—called system logs—and represents this information as graphs. Imagine a graph where each node is a file or a program running on a computer, and lines connect these nodes to show how they are related. This makes it easier to see patterns that might indicate a threat.
But what makes MEGR-APT special is its use of artificial intelligence, specifically a type called graph neural networks. These networks learn from data and can predict which patterns are suspicious. The system extracts small parts of these graphs and checks them against known attack patterns, quickly identifying potential threats without using a lot of memory.
The researchers tested it with real-world data and found it to be highly effective. It used much less memory than other systems and was just as accurate and fast. This means it can handle large amounts of data and still find threats quickly, making it a practical tool for keeping our digital world safe.
Read the full research published in the journal IEEE Transactions on Information Forensics and Security.
Learn more about Concordia’s Department of Computer Science and Software Engineering and Concordia Institute for Information Systems Engineering