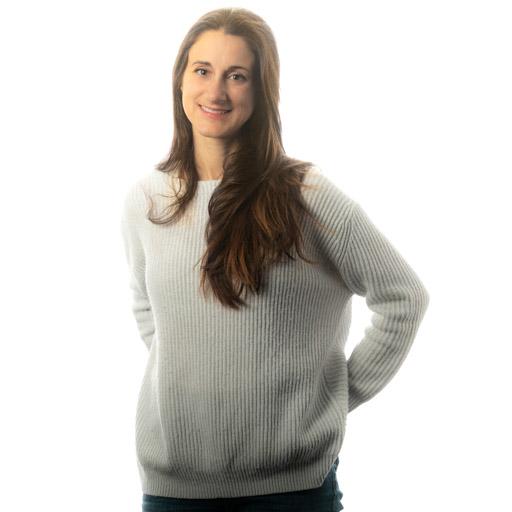
Mélina Mailhot, PhD
- ASA, ACIA
- Associate Professor, Mathematics and Statistics
Are you the profile owner?
Sign in to editContact information
Email:
Biography
Education
Ph.D.: Université Laval, Canada 2012
Research interests
Actuarial Science, Risk Theory, Dependence Modeling, Risk Measures, Optimization
Teaching activities
Mathematics of Finance
Loss Models
Investment Mathematics
Risk Theory
Risk Measures
Research activities
Publications
C. Araiza I.*, F. Godin, M. Mailhot (2021). Tweedie double GLM loss triangles with dependenc within and across business lines. European Actuarial Journal, in press. N. Beck*, E. Di Bernardino, M. Mailhot (2021). Semi-parametric estimation of multivariate extreme expectile. Journal of Multivariate Analysis, doi.org/10.1016/j.jmva.2021.104758 K. Herrmann, M. Hofert, H. Mailhot (2020). Multivariate geometric tail- and range-value-at-risk. ASTIN Bulletin, 50 (1), p.-265-292, doi:10.1017/asb.2019.31 N. Beck*, C. Genest, J. Jalbert, M. Mailhot (2020). Predicting extreme surges from sparse data using a copula-based hierarchical Bayesian spatial model. Environmetrics. doi.org/10.1002/env.2616 K. Herrmann, M. Hofert, H. Mailhot (2018). Multivariate Geometric Expectiles. Scandinavian Actuarial Journal, 7, p. 629-659. N. Beck*, M. Mailhot (2018). A Consistent Estimator to the Orthant-Based Tail Value-at-Risk. ESAIM: Probability and Statistics, 22, p. 163-177. M. Mailhot, M. Mesfioui (2016). Multivariate TVaR-Based Risk Decomposition for Vector-Valued Portfolios. Risks, 44(4). B. Kchouk*, M. Mailhot (2016). Reciprocal Reinsurance Treaties Under an Optimal and Fair Joint Survival Probability. Variance. H. Cossette, M. Mailhot, E. Marceau, M. Mesfioui (2015). Vector-valued Tail Value-at-Risk. Methodology and Computing in Applied Probability, p.1-22. H. Cossette, M.-P. Côté, M. Mailhot, E. Marceau (2014). A note on the computation of sharp numerical bounds for the distribution of the sum, product or ratio of dependent risks. Journal of Multivariate Analysis, 130, p.1-20 H. Cossette, M. Mailhot, E. Marceau, M. Mesfioui (2013). Bivariate lower and upper orthant Value-at-Risks. European Actuarial Journal, 3 (2), p.321-357. H. Cossette, M. Mailhot, E. Marceau. (2012). TVaR-based capital allocation for multivariate compound distributions. Insurance : Mathematics and Economics, 50 (2), p.247-256. J.-F. Quessy, M. Mailhot (2011). Asymptotic power of tests of normality under local alternatives. Journal of Statistical Planning and Inference, 141, p.2787- 2802
Participation activities
Invited Presentations
Online International Conference in Actuarial Science, Data Science and Finance, 2020 Statistics Seminar, Laval University, 2020 Groupe d’étude et de recherche en analyse de décision, University of Montréal, 2019 Annual Meeting of the Canadian Institute of Actuaries, Montreal, 2019 Annual Meeting of the Statistical Society of Canada, University of Calgary, 2019 International Conference on Statistical Distributions and Applications, Grand Rapids, 2019 Annual Meeting of the Statistical Society of Canada, McGill University, 2018 CRM Thematic Semester, McGill University, 2017 Workshop in Insurance Mathematics, Waterloo University, 2016 International Conference on Statistical Distributions with Applications, Niagara Falls, 2016 Annual Meeting of the Statistical Society of Canada, Brock University, 2016 CRM Seminar, Université Sherbrooke, 2015 CRM Seminar, Université de Montréal, 2015 Lecture,“Actuariat et société”, Université du Québec à Montréal, 2015 Conference, National Association of Students in Actuarial Science, 2015 Lecture “Actuariat et société”, Université du Québec à Montréal, 2014 Conference, Industrielle Alliance, 2012 Seminar, Université Concordia, 2012 Seminar, Université de Montréal, 2012
Weekly Seminars
August 11th
Speaker: Nicholas Beck
Title: Predicting extreme surges from sparse data using a copula-based hierarchical Bayesian spatial model
Abstract:
A hierarchical Bayesian model is proposed to quantify the magnitude of extreme surges on the Atlantic coast of Canada with limited data. Generalized extreme value distributions are fitted to surges derived from water levels measured at 21 buoys along the coast. The parameters of these distributions are linked together through a Gaussian field whosemean and variance are driven by atmospheric sea-level pressure and the distance between stations, respectively. This allows for information sharing across the original stations and for interpolation anywhere along the coast. The use of a copula at the data level of the hierarchy further accounts for the dependence between locations, allowing for inference beyond a site-by-site basis. It is shown how the extreme surges derived from the model can be combined with the tidal process to predict potentially catastrophic water levels.
August 4th
Speaker: Sébastien Jessup
Title: Factors and projecting the behaviour of extreme rainfall through time
Abstract:
Factors named areal reduction factors are used in environmental engineering to extrapolate rainfall over a delimited area from data at a specific weather station, known as point rainfall. Thy depend on caption areas, duration of precipitation, and return periods. We look at some mathematical properties of ARFs and attempt to use them to study extreme rainfall projections from 2006 to 2100.
July 15th
Speaker: Magali Goulet
Title: Neural Networks in Insurance
Abstract:
The insurance industry is a goldmine of data. It is also a world of uncertainties, especially when it comes to pricing and risk assessment. Considering the vast amount of available data, a technique that can handle it is Neural Networks. It is mostly known to be used in speech and image recognition, but it is used for many other tasks. It is a machine learning technique but even more specifically, it involves deep learning. The idea is that we can pass a lot of input/output pairs of data to train the model so that it can make predictions using new sets of inputs. It can deal with a very large numbers of covariates, which is also an interesting feature in insurance.
July 7th
Speaker: Éloi D'Amour Bizimana
Title: Multivariate extreme analysis – Where to look (A Literature Review)
Abstract:
When analyzing a univariate dataset, an extreme is either a maximum or a minimum. That is, the only two directions we can consider are “up” (towards positive infinity) and “down” (towards negative infinity). When considering two dimensions, however, the amount of directions we can consider are infinite. Indeed, any unit vector in R2 describes a rotation of the dataset which allows us to “look” at the data differently, with the traditional direction being e. Given a direction vector, a new definition of extremes is prescribed. This allows for a fully non-parametric approach to multivariate extreme detection which can be extended to the n-variate case. A copula approach is defined as well. Various case studies are performed and show that data rotation can lead to much more precise results.
June 30th
Speaker: Fidence Munyamahoro
Title: Insurance Applications of Mixture-Type Copulas
Abstract: Modelling dependence of financial losses enabling insurers, reinsurers, and other Risk modelersto analyze the probability and severity of economic losses from extreme loss events. Theimportance of copulas has been growing because of their applications in several fields of research. Thecopula is a flexible modeling tool that allows construction of multivariate models. Copula isbecoming very popular model to model dependencies and it flexibly allows the construction ofmultivariate distribution through any univariate marginals. Mixture models have been widelystudied and applied to various application areas. We are interested with the application ofmixture copula models such as convex combination and parameter mixing of copula models.Spearman’s rho and Kendall’s tau are two most commonly used nonparametric measuresof association for two random variables. However, they came with some amount of limitationsfor bivariate (or multivariate) distributions. Mixture copula with well-chosen mixing parameterincreases the range of these measures for many types of copula models. We estimatedvalues of these dependence measures for convex combination of Gumbel and Claytoncopulas. The mixture copula provides increased flexibility to adapt to different dependencestructures, since it unites the diverse features of the enclosed copulas.
June 16th
Speaker: Gabriel Haeck
Title: Macroeconomic Variables in Option Pricing
Abstract:
Generalized Autoregressive Conditional Heteroskedasticity (GARCH) models have been the most prominent tool in Financial Time Series for option pricing.
Based on recent literature, when acknowledging the stochastic nature of volatility, one must consider the expected distribution of future volatility. As such, option prices may depend on other variables not perfectly correlated to stock returns. In this study, we consider macroeconomic variables (e.g. business conditions) as a proxy for the missing variables.
Thus, we consider a Multivariate GARCH model that first accounts for market dynamics through the macroeconomic variables, then accounts for individual stock dynamics, where the stock dynamic is dependent on the market dynamic and where stock volatility revolves around a fundamental volatility driven by the macroeconomic variable.
June 9th
Speaker: Nahid Sadr
Title: Distortion Functions; Multivariate and Extremes
Abstract:
Distortion functions have been used in the pricing of insurance contracts for a long time. It is used to transform the probabilities of the loss distribution to another probability distribution by re-weighting the original one. The essential idea of distorted insurance pricing is not only to overvalue outstanding potential losses but also to underweight small claims. This approach provides a risk-adjusted premium, which always exceeds the net premium. Parametric models for multivariate distributions can be proposed based on distortion functions, that permit to generate new families of multivariate distribution functions. To evaluate the quality of candidate distortion parameters in the estimation procedure, PI (Proximity Indicator) is introduced. We finally get parametric representations of multivariate distorted distribution functions which are illustrated on real data examples.
These representations will further be used to propose univariate and bivariate climate models for annual temperature and precipitation extremes.
June 2nd
Speaker: Emily Wright
Title: Strongly Connected Node Reliability
Abstract:
Network reliability has been widely studied for over 70 years. With networkds playing such a large part of modern-day life, it is important to understand the reliability of a network. Various models of networkreliability exist, but in this talk, we introduce a new model Strongly Connected Node Reliability. Consider a digraph D on n vertices and m arcs where the vertices operate independently with probability p in [0,1]. The Strongly Connected Node Reliability of D, denoted scNodeRel(D,p), is the probability that the subdigraph induced by the operational vertices is strongly connected. We present results on the analytic properties of the reliability polynomial on the interval [0,1] including inflection points and intervals of increase and decrease. We also discuss the existence of optimal digraphs for this reliability model and compare the results to the undirected model, the Node Reliability of a graph.
May 26th
Speaker: Haoyu Zhang
Title: TuckER with Background Knowledge
Abstract:
Knowledge graphs are a way to represent a large amount of relational facts coming from real word knowledge. Since that knowledge is generally incomplete, a vast research area, known as Link Prediction is devoted to infer possible unknown facts based on the existing ones. We focus on a recent state-of-the-art linear model called TuckER that was introduced for the task of link prediction by Balazevich et al. (Balazevic et al., 2019). In this project,we propose ways to incorporate background knowledge about symmetric and asymmetric relations. We show that our model performs better than the TuckER model on those relations while requiring half of parameters.
May 19th
Speaker: Nicholas Beck
Title: Semi-parametric Estimation for Multivariate Extreme Expectiles
Abstract:
This presentation focuses on semi-parametric estimation of multivariate expectiles for levels of significance α ≈ 1.Multivariate expectiles and their extremes have been the focus of plentiful research in recent years. Inparticular, it has been noted that due to the difficulty of estimating these values for elevated level of α analternative reformulation of the underlying optimization problem would be necessary. However, in such ascenario, estimators have only been provided for the limiting cases of extreme tail dependence: independenceand comonotonicity. In this paper, we extend the estimation of multivariate extreme expectiles (MEEs) byproviding a consistent estimation scheme for random vectors with any arbitrary dependence structure.Specifically, we show that if the upper tail dependence function, tail index, and tail ratio can be consistentlyestimated, then one would be able to accurately estimate MEEs. The finite-sample performance of thismethodology is demonstrated using both simulated and real data.
May 12th
Speaker: Roba Bairakdar
Title: Simulation-based earthquake insurance risk calculation
Abstract:
The insurance risk arising from catastrophes such as earthquake risk exposure is one of the components of the Minimum Capital Test (MCT) for federally regulated property and casualty insurance companies. The current guidelines of the Office of the Superintendent of Financial Institutions Canada (OSFI) expects the insurer to meet a test of financial adequacy for a 500-year return period country-wide earthquake based on equal weights for eastern and western Canada. In this project, a simulation based approach is used in which losses and insurance claim payments are calculated based on earthquake hazard maps of Canada. Building occupancy classifications and their respective damage probability matrices are combined with the earthquake insurance market penetration to compare the risk in eastern vs western Canada, which shows an imbalance, we per our most recent calculations.
May 5th
Speaker: Sébastien Jessup
Title: Modelling of unearned premium risk with dependence
Abstract:
Unearned premium, or more particularly the risk associated to it, has only recently received regulatory attention. Losses linked to unearned premium occur after the evaluation date for policies written before the evaluation date. Given that an inadequate acquisition pattern of premium and approximate modelling of premium liability can lead to an inaccurate reserve around unearned premium risk, an individual nonhomogeneous loss model including cross-coverage dependence is proposed to provide an alternative method of evaluating this risk. Claim occurrence is analysed in terms of both claim seasonality and multiple coverage frequency. Homogeneous and heterogeneous distributions are fitted to marginals. Copulas are fitted to pairs of coverages using rank-based methods and a tail function. This approach is used on a recent Ontario auto database.
Apr. 28th
Speaker: Mélina Mailhot
Title: Distribution-based Multivariate Range Value-at-Risk and Extreme Values
Abstract:
We are currently in a transition period for insurance companies' regulation, moving from country-specific guidelines to the new IFRS 17 framework. The changes are related to the financial reporting and calculation of reserves. Range Value-at-Risk (RVaR) is a risk measure that averages values between bounds (quantiles). This characteristic allows for the representation of high levels of risks, up to a specified limit, which is convenient for infinite mean distributions, for example. The multivariate cases will be defined. Specific results of RVaR in the extreme value framework will be presented, considering GEV and GPD distributions, as well as asymptotic results for extreme confidence levels. An empirical estimator will be presented with numerical illustrations.