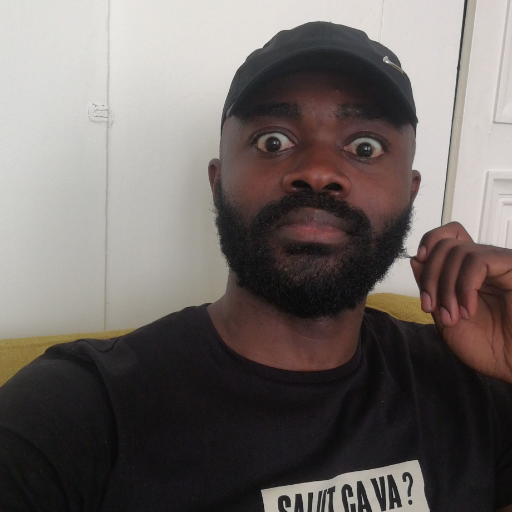
Elvis Dohmatob, PhD
- Associate Professor, Computer Science and Software Engineering
Are you the profile owner?
Sign in to editResearch areas: artificial intelligence, machine learning, learning theory, algorithms, neural networks, neural scaling laws, adversarial robustness, algorithmic bias, optimization, determinantal point processes
Contact information
Email:
Availability:
I'm always looking for passionate graduate students. If you are thinking about a doing PhD in fundamental aspects of ML (theoretical and algorithmic), and you genuinely feel that your profile matches the kind of research I do (see my papers), don't hesitate to drop me an email. Note that I will not reply to random supervision requests!
Biography
I am a researcher working on various topics in artificial intelligence (AI) and machine learning (ML), with a theoretical flavor. I joined Concordia as a professor in 2024. I'm also an affiliate faculty at the Mila Institute. Prior to Concordia, I held positions at INRIA (Paris, France), Criteo (Paris, France), and FAIR/Meta (Paris, France).
My current research agenda focuses around the following themes:
- Learning Theory (neural scaling laws, robustness, model collapse, etc.),
- Neural Networks (attention, associative memories, etc.),
- Representation Learning,
- Trustworthy AI/ML (algorithmic bias, adversarial robustness, etc.),
- Optimization (convex, discrete, etc.)
Publications
2025
- A. Subramonian, S. Bell, L. Sagun, E. Dohmatob, "An Effective Theory of Bias Amplification", In The Thirteenth International Conference on Learning Representations (ICLR), 2025
- Y. Feng, E. Dohmatob, P. Yang, F. Charton, J. Kempe, "Beyond Model Collapse: Scaling Up with Synthesized Data Requires Reinforcement", In The Thirteenth International Conference on Learning Representations (ICLR), 2025
- R. Bayat, M. Pezeshki, E. Dohmatob, D. Lopez-Paz, P. Vincent, "The Pitfalls of Memorization: When Memorization Hurts Generalization", In The Thirteenth International Conference on Learning Representations (ICLR), 2025
- E. Dohmatob, Y. Feng, A. Subramonian, J. Kempe "Strong Model Collapse", In The Thirteenth International Conference on Learning Representations (ICLR), 2025
2024
- E. Dohmatob, Y. Feng, P. Yang, F. Charton, J. Kempe, "A Tale of Tails: Model Collapse as a Change of Scaling Laws", In International Conference on Machine Learning (ICML), volume 235 of Proceedings of Machine Learning Research, 2024
- E. Dohmatob, Y. Feng, J. Kempe, "Model Collapse Demystified: The Case of Regression", In Advances in Neural Information Processing Systems (NeurIPS), volume 37, 2024
- V. Cabannes, E. Dohmatob, A. Bietti, "Scaling Laws for Associative Memories", In The Twelfth International Conference on Learning Representations (ICLR), 2024
- E. Dohmatob, M. Scetbon, "Precise Accuracy / Robustness Tradeoffs in Regression: Case of General Norms", In International Conference on Machine Learning (ICML), volume 235 of Proceedings of Machine Learning Research, 2024
- E. Dohmatob, "Consistent Adversarially Robust Linear Classification: Non-Parametric Setting", In International Conference on Machine Learning (ICML), volume 235 of Proceedings of Machine Learning Research, 2024
Complete List
Are you the profile owner?
Sign in to edit
Took 46 milliseconds