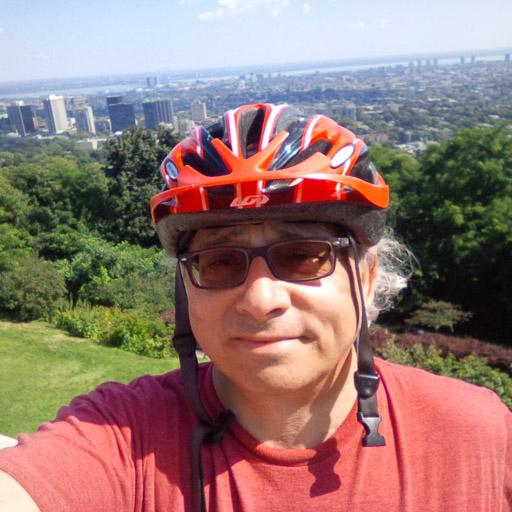
Dr. Krzysztof Dzieciolowski, PhD
- Part-time Faculty, Supply Chain and Business Technology Management
Are you the profile owner?
Sign in to editResearch areas: Social networks, predictive models, text mining, big data, fuzzy logic matching, time series, geospatial analytics
Contact information
ORCID:
Biography
Biography
Dr. Dzieciolowski’s career combines over 30 years of experience in data science and leadership roles in the Canadian telecommunication industry with teaching and research, as a part-time faculty member at John Molson School of Business in Montreal.
In his professional career, he led Data Science and Modelling teams in Rogers and Bell Canada, where he developed predictive models and analytical solutions for their key business problems.
Currently, Dr Dzieciolowski serves as a Chief Analytics Officer at Daesys Inc., the AI startup company in Montreal developing Machine Learning Operations (MLOps) platform for Machine Learning models.
At the John Molson School of Business (JMSB), Dr. Dzieciolowski researches and teaches courses in data mining, statistics, data science and analytical software to undergraduate and graduate students. He has built synergies between industry and academia and obtained internship funding for students of Montreal universities.
In 2016, he's developed and launched a highly successful, joint Certification in Business Analytics and Predictive Modelling between JMSB and SAS – the world’s leader in data science software. Since then, hundreds of undergraduates and graduates students received the Certification to enhance their career and professional opportunities upon graduation.
Dr. Dzieciolowski introduced novel methods of teaching and experiential learning in the classroom, leveraging support of SAS software and its learning infrastructure. He has advised and guided the development of new data science programs such as Data Intelligence Minor, M.Sc. in data science and B.Sc. in data analytics. He advised M.Sc. and PhD students in data science and machine learning.
Dr. Dzieciolowski's research interests focus on statistics and data science methods; in particular, on machine learning models diagnostics and explainability, predictive models techniques, multivariate analysis, forecasting, social networks and text analytics. He was distinguished with the Concordia University Part-Time Faculty Association (CUPFA) Special JMSB Research award for the project in Big Data Analytics.
He is an active promoter of data science and AI methods and has been a frequent speaker at the academic and industry conferences in North America and Europe.
Dr. Dzieciolowski obtained M.Sc. in mathematics from Warsaw University in Poland and Ph.D. in statistics from Queen’s University in Kingston, Canada.
Teaching activities
Data Mining Techniques - BSTA 478/678
The objective of the course is to introduce basic concepts and techniques of Data Mining and its applications in business. In recent years, Data Mining, often also referred to as Data Science, has become one of the most dynamically growing areas of business knowledge discovery field. Proliferation of computing resources, Internet, social networks, mobile communication with the resulting deluge of data, often called -Big Data, has motivated companies to turn data into an asset for decision-making and used gain competitive advantage.
The course focuses on supervised and unsupervised data mining methods, their underlying principles and applications for structured data as well as unstructured data such as text. The data mining techniques introduced throughout the course include regression models and learning algorithms such as k-nearest neighbours, naïve Bayes, classification and regression trees, support vector machine, neural networks, discriminant analysis, association rules, cluster analysis and text mining.
Statistical Software for Data Management and Analysis - BSTA 445/645
The objective of the course is to gain a basic understanding of the concepts, principles and techniques of data management and statistical analyses through the use of Statistical Software. The focus of this course is on practical aspects of data management and statistical analysis with the help of Statistical Analysis System (SAS) – widely used by business, government and academia. In the process of familiarizing with SAS, principal attention will be given to data management techniques related to handing of data storage, information retrieval, variable and row transformation, data cleansing, file handling and preparation of data for analytics, visualization, statistical analysis and reporting. The course helps students understand methods of handling big data through the use of principles of efficient programming. Through hands-on approaches, students learn how to design and execute programs for the data-intensive processes.
Managerial forecasting - MBA 677
The course focuses on the theory and applications of the most used methods of business forecasting, including smoothing, differencing, exponential smoothing, decomposition, linear regression models with varying data patterns, as well as ARMA, ARIMA and seasonalARIMA models. Some more advanced methods are explored at the end of the course.Key features of time series data are explored. The course also introduces tools needed to evaluate many forecasting scenarios: identifying benchmark forecasting methods, diagnostic checking to determine if a method has adequately utilized the available information in the data, techniques for computing prediction intervals, and methods for evaluating forecast accuracy. Practical usage of the methods and techniques are demonstrated through case assignments and a term project, using business and economic datasets.Students are expected to have a basic knowledge of statistical concepts to follow this course.
Managerial Analytics - MBA 643
This course emphasizes the development of analytical skills needed to work effectively in a business environment. It introduces the basics of knowledge discovery from big data, business analytics and predictive modelling for data driven decision-making and policy formation. Topics include exploratory data analysis, statistical analysis, and modelling, forecasting and data visualization. Practical usages of the methodologies are demonstrated via projects and case analysis based on big data from various functional areas of business.
Research activities
2016
Academic - industry research sponsor and facilitator 2000-2022:
- sponsoring multiple data science projects between universities and Bell Canada and Rogers
- funding students' internships for students of Montreal universities (McGill, Concordia, University of Montreal)
- Collaborating on text mining models with Y. Bengio (University of Montreal)
Publications
1. Ningsheng Zhao, Jia Yuan Yu, Trang Bui, Krzysztof Dzieciolowski, 2024. Correcting Biases of Shapley Value Attributions for Informative MachineLearning Model Explanations, CIKM '24: Proceedings of the 33rd ACM International Conference on Information and Knowledge Management, Pages 3331–3340,
https://doi.org/10.1145/3627673.3679846
2. Ningsheng Zhao, Jia Yuan Yu, Krzysztof Dzieciolowski,Trang Bui,
Error Analysis of ShapleyValue-Based Model Explanations: An Informative Perspective, SAIV 2024, Part ofthe book series: Lecture Notesin Computer Science ((LNCS,volume 14846)), Included in thefollowing conference series: InternationalSymposium on AI Verification
3. K. Dzieciolowski, Y. P. Chaubey, F. Nebebe and D. Sen, 2009, A Semi-Bayesian Approach for Estimation of Joint Distribution from Marginal Distributions, International Journal of Statistical Sciences ISSN 1683–5603, Vol. 9;
5. K. Dzieciolowski, W.H. Ross, (1990), Assessing Case Influence on Confidence Intervals in Nonlinear Regression, Canadian Journal of Statistics, v18, 2, 127-139
Conferences and presentations
Big Data Analytics, Data Mining and Computational Intelligence-IADIS, Porto, Portugal 2023
Towards Explainable Machine Learning Operations (MLOps)
Big Data Analytics, Data Mining and Computational Intelligence-IADIS, Lisbon, Portugal 2022
Classifier Rank - A New Classification Assessment Method
SAS Global Forum, Dallas, USA, 2019
Explaining Machine Learning Models
Big Data Analytics, Data Mining and Computational Intelligence-IADIS, Porto, Portugal 2019
Explaining Black-box Machine Learning Models
SAS Global Forum, Denver, 2018
K. Dzieciolowski, "Creating a Successful Data Science Program – A Joint Academic and Industry Perspective"
SAS Global Forum - Orlando, USA, 2017
K. Dzieciolowski, "Fuzzy Matching and Predictive Models for Acquisition of New Customers"
Big Data and Data Mining, JMSB, Concordia, Montreal, Canada 2016
http://www.concordia.ca/content/shared/en/events/jmsb/2016/03/15/big-data-and-data-mining.html
European Conference in Data Mining, Las Palmas, Spain 2015
Predicting Customer Attrition with Markov Chains
European Conference in Data Mining, Lisbon, Portugal, 2014
Predictive Probabilities in Marketing
International Association for Development of the Information Society, IADIS, Lisbon, Portugal 2012
Why Data Mining is Difficult to Learn? And What Can We Do to Make It Easier?
International Association for Development of the Information Society, Amsterdam, Netherlands 2008
Data Mining in Marketing Acquisition Campaigns
MITACS Quebec Interechange on Data Mining, Montreal, Canada 2003